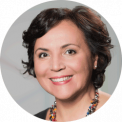
The challenge is to encourage cross-functional work and to manage digital, data and AI in a coherent way.
Samia Bendali-Amor
The health crisis has put the spotlight on the interest of digital technology and, in particular, the efficiency of data-AI technologies. In France, its deployment in companies is at its restructuring stage. In fact, AI projects are deeply transformative. Their success requires organizational changes, working methods changes, adapted technological choices and an ethical and legal framework definition. It requires the integration of these transformation projects in the global strategy of the company and an adapted support to contribute to the creation of the augmented company
The health crisis has accelerated the recognition of data-AI benefits
The emergence of the digital age has undoubtedly contributed to the fight against COVID-19 and to the reduction of the impacts of the unprecedented health crisis we are going through. Among the various technologies of this new era, aata-AI or Data Intelligence has contributed to the assessment, projection, analysis and management of the pandemic. There are many examples. The best known are those related to pandemic assessment and medical diagnosis:
- Mapping of the pandemic: Big Data combined with Geographic Information Systems (GIS) have enabled the development of dynamic maps to visualize the extent of the pandemic, by performing spatio-temporal analyses from several different sources of information.
- Predicting the evolution of the pandemic: Data Science has performed predictions of the evolution of virus spread at the regional, national and global levels and its projection in time.
- Medical diagnosis: facial recognition coupled with thermal capture is used to identify people with high temperatures (in transportation for example). The implementation of telemedicine platforms has enabled the first diagnoses to be carried out and patients to be sorted.
The pandemic has had catastrophic consequences on our economy and our society. But, in the midst of the emergency, we had to deal with it and find innovative solutions. And, ironically, the crisis has also had positive impacts: it has accelerated innovation and reinforced the use of digital tools, including data-AI. Although this technology has been gaining interest for a few years, it continues to intrigue, question and raise issues of ethics, responsibility and privacy.
The advantages of data-AI and its capabilities of detection, prediction and natural interaction with humans (automatic natural language processing) have become apparent. This transformative field opens the door to new innovative services.
However, its use in companies, especially those that inherit a significant "legacy", in other words an information system based on outdated technologies and/or a heavy functional heritage, remains at the exploration stage. A study conducted in 2018 by TCS & IDC on a panel of 300 French companies shows that only 36% of them have already implemented AI tools, while 16% indicate that they intend to develop AI projects within the next 1 to 3 years [TCS][1]. Companies are still in the structuring phase, which leads them to face several new challenges.
Define the new organisational schemes of the augmented enterprise
Organizations need to transform themselves in order to federate initiatives, pool skills, and break down the barriers between departments by bridging the gap between business and IT departments. The challenge is to encourage cross-functional work and to manage digital, data and AI in a coherent way.
Above all, the transformation implies a cultural and human change at all company levels. New skills will have to be integrated: data scientists, chatbot coaches, psy-designers in charge of designing AI interfaces or ethicists responsible for the governance of decisions made by AI tools. New jobs are emerging and we will have to face the shortage of these new profiles on the market. Training, acculturation, acquisition and partnership strategies, including startups, will be crucial.
This hybrid enterprise transformation, requiring the management of existing information systems while creating new intelligent systems - knowing that some can interact with others - contributes to the construction of an augmented enterprise [CIGREF report][2] through intelligent collaboration capabilities between man and machine.
The pandemic has had catastrophic consequences on our economy and our society. But, in the midst of the emergency, we had to deal with it and find innovative solutions.
Transforming working methods: Agility as a synergy catalyst
Cross-functional collaboration is crucial to the successful integration of data-AI in companies: business lines, IT specialists, statisticians and data scientists must be able to work together.
Since data-AI culture is not sufficiently mature in companies, working in agile mode allows us to move forward in small steps (Think Big, Start Small and Show Value Fast) and to capitalize on failures as soon as possible. We are witnessing the emergence of MLOps (Machine Learning Operations) and DataOps (Data Operations) methods, an adaptation of DevOps[3] to data-AI projects.
The challenge is to create multi-disciplinary teams and collaborative spaces (data lab, fab lab, etc.) to catalyze synergies and converge projects by aligning all stakeholders through a shared methodology and a common culture.
Make technology choices adapted to business use cases
Data-AI is a broad field that covers several technologies: robotics, natural language processing, pattern recognition, machine learning, deep learning, predictive analysis, uncertain reasoning, knowledge representation, semantic web, multi-agent systems... These different technologies allow the creation of a multitude of business use cases such as virtual assistance, automatic detection of breakdowns or fraud, predictive marketing... The choice of technological solutions cannot be made without taking into consideration the business use cases to be implemented.
Companies should also consider the question of technology choice - "make-or-buy" - and seriously consider defining a strategy around open source. Indeed, the GAFAs have developed many open source technical platforms and frameworks[4]. They are ahead of the game in this area since their business model has always been based on data. Using their tools allows you to get started quickly, to check the relevance of use cases and to evaluate the benefits at the lowest cost.
Defining an ethical and legal framework to keep technology under human control
Data-AI relies on the processing of large amounts of data, in particular for machine learning and prediction algorithms. Consequently, it raises many questions about the use of this data while respecting privacy and individual freedoms, and triggers mistrust.
It also raises transparency and algorithm control issues: how to guarantee that these learning algorithms do not generate inequalities or exclusion? How to manage the issue of responsibility for AI-based decisions? A collective challenge must be met to ensure that these new tools are under human control, at the service of humans, respecting transparency and responsibility [CNIL][5].
The challenges of data-AI[6] are numerous, and its success will depend heavily on how well it is integrated into the company's overall strategy, its coherence with the digital transformation program underway, the governance of the data, and the inherent change management
Extract from our white paper : Challenges and advancements in the era of data and artificial intelligence