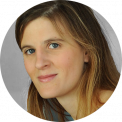
Telecoms are indeed among the most data-rich industries.
Coralie Muratet
Organizations sometimes find themselves confronted with technological innovations which question their strategies and operating methods. Just like digital, (Big) Data and AI are among the innovations that change the rules of the game and push companies to reinvent themselves. While most telecom operators have embarked on this path, they have not necessarily managed to model with a high degree of confidence the value, in the sense of financial benefits, linked to data and AI.
A favorable context for value creation
Although neither of these technologies is particularly new, their continuous progress and the concomitant drop in the costs associated with their use, marked by an acceleration since the end of the last decade, have made their democratization possible. AI algorithms have indeed gained in performance by feeding on the ever-increasing volumes and variety of digital data, especially unstructured data, covering almost all areas of human activity.
The efficiencies of the hardware and software ecosystems that enable Big Data processing are now available to enterprises, which was once reserved for the research world: computing power at the (un)scale of AI, both cheaper and more powerful. While the cost of training a neural network to recognize images was as high as $1,000 on a public cloud in 2017, it was only $10 just two years later, in 201917.
And this trend continues. Data explosion is also unlikely to end: in the next three years, more data is expected to be generated than in the last 30 years18.
In telecoms, the opportunity is huge
Telecoms are indeed among the most data-rich industries. According to IDC, 9% of all enterprise data stored in the world was created by telecoms and utilities19. Just like energy or water players, telecom operators provide essential services.
Their customer bases can therefore represent significant fractions of the population and businesses in a given territory. Their data is therefore likely to have a certain statistical representation.
Fixed-mobile convergence, omnichannelity and diversification into new services are also increasingly varied: operators' customers can often use their mobile or the Internet at the same time, watch IPTV, use mobile financial services and contact their customer service department through multiple channels (on the Internet, in a store or by telephone, in particular); each time, they generate data.
However, it is mainly because the networks and their equipment are the transit points for all digital exchanges, that the volumes and variety of data in the hands of operators are potentially gigantic. Of course, not all this data is intercepted or stored, and the data that is intercepted or stored is often anonymized and aggregated.
But the methods for collecting and analyzing this data - fortunately very well regulated in most countries - have existed for a long time, such as deep packet inspection (DPI). Add to this the arrival of 5G and its promise of IoT data development, and the prospects for data valuation by operators quickly become dizzying.
The evaded question of value destruction
It is true that Data and AI arouse the fear of seeing certain jobs become obsolete, including - and this is the novelty - so-called "skilled" jobs, but operators were quickly relieved to realize that they were much less of a threat to them than digital was: they do not entail the risk of disintermediation, the appearance of new over-the-top entrants and, ultimately, the loss of revenue. This difference could be major in the speed with which operators will seize Data and AI.
The thorny issue of profitability
There are many examples of successful applications of data and AI in areas critical to operators: from fraud detection to planning network deployments to ensure the most profitable investments, from optimized management of network resources to increasingly personalized direct marketing campaigns.
But these concrete examples are not enough to estimate the profitability of Data and AI on a company-wide scale. And for good reason: once operators have identified the priority Data and AI use cases for their various businesses, they should ideally make several cross-functional expenditures to create the conditions for deploying these uses.
In particular, they need an infrastructure that allows for collection and integration (sometimes in real time), storage (if not centralized, then at least avoiding duplication and favoring the cross-referencing of sources) and sufficiently rapid processing (increasingly deported to the edge of networks, or even embedded).
This infrastructure must be adapted to the governance of their data and its security, in compliance with regulations, particularly those concerning confidentiality and sovereignty. These investments potentially benefit all of the operators' data and AI use cases, and their costs should therefore ideally be shared between all the projects and entities that benefit from them. However, infrastructure expenses can represent a significant portion of operators' total Data and AI expenses, especially since models must be continually trained with new data to remain efficient.
At the same time, operators must spend money on transforming their organizations, often going so far as to create entities dedicated to data and AI. In particular, they have to finance the required transformation of their employees, their acculturation, their skills upgrading or their evolution towards new professions, and recruit the skills they lack and which are often expensive, because the context is one of shortage.
Moreover, the exploratory nature of some of the projects around the data, the uncertainty of obtaining exploitable results, the fluctuating time of learning and the quality of the data itself make the cost of this common technical base and the expected value difficult to predict.
Finally, operators' spending is rarely confined to one technology or technique: the use of AI is often coupled with automation, especially in telecoms. Moreover, technology is only one lever among others to explain the creation of value: beyond the machine, there is of course and above all the human factor, the interactions between one and the other, the data without which the machine could not learn and many other explanatory factors. It is therefore complex, if not impossible, to use AI or data alone to enhance their individual contribution to the benefits of a company or a specific project.
Proving value by example
Even if the international operators communicating on the cost of their investments in the field of data and AI, as well as on the expected and actual returns of these investments, can be counted on the fingers of one hand, it seems that the efforts are beginning to bear fruit for some. At least, this is what the first of them to have invested significantly in the field are reporting.
For example, Vodafone points to operational efficiencies equivalent to 3,500 FTEs over the past 2 years thanks to robotics and AI20 - no small feat for a group of 93,000 employees.
Telefónica21, for its part, launched Luca in 2016, a Big Data subsidiary aimed at enterprise customers, which has since become a Big Data and AI subsidiary. Shortly afterwards, Telefónica21 embarked on an ambitious strategy to create a "fourth platform" of cognitive intelligence, Aura, based on natural language, which was to become the orchestra conductor of an organization that until then had been structured around three components: physical infrastructure, information systems, and products and services. Telefónica's financial communication didn’t say much about the marginal cost of Aura, except that 48 billion euros would have been spent from 2012 to 2017 to finance the prerequisites for its creation. Little do we know about the revenues or cost savings that could be attributed to it; Telefónica preferring to communicate on the benefits of digitalization in general, to which it associates Big Data and AI, among other technologies. At the end of 2019, however, Aura claimed 3 million monthly active users and a deployment within 1000 use cases.
Even if the examples and figures are still few and far between, they constitute a precedent that has emerged quite recently. However, if the pioneering operators of data and AI start to see a tangible gain, and know how to express it on the scale of their company as a whole and not only of singular projects, it is certain that those who are less advanced will want to follow suit.
Data and AI spending continues
According to Omdia22, more than 50% of operators plan to increase their spending on AI tools and data lakes in 2021, despite (or because of) the pandemic. Just as digitalization accelerated at a rapid pace in 2020 - offsetting years of cautious investment by operators in the field - so did the use of AI, to enhance engagement with consumer customers lacking access to traditional human channels (stores and contact centers, in particular).
A movement is also beginning to gain momentum among operators: the migration of part of their data and IS to the cloud and the few solutions on the market, mainly American. However, the issues of security, confidentiality and data sovereignty, to name but a few, have long been very strong brakes on this movement. But with the development of data and AI, other considerations seem to prevail.
First, the technical strategies adopted earlier by operators to extract value from their data, such as on-premise Hadoop architectures, have revealed their limitations and hidden costs. Second, the cloud and its promise of elastic storage and computation have simplified access to resources and made much of their costs variable.
While the cloud is helping to control the costs associated with data and AI, the question remains as to the exact value of these technologies to telecom operators.
1 Exemple de classification par réseau de neurones à convolution ResNet50. Source : Ark Invest, The Cost of AI Training is Improving at 50x the Speed of Moore’s Law: Why It’s Still Early Days for AI, 2020
2 Source : IDC, Worldwide Global DataSphere Forecast, 2020–2024: The COVID-19 Data Bump and the Future of Data Growth, 2020
3 Source : IDC, Data Age 2025, 2018 14 Source : rapport annuel 2020, Vodafone Group Plc
4 Sources : communiqués de presse, rapport annuel et blog Telefónica, 2016 à 2019
5 Source : Omdia, ICT Enterprise Insights 2020/21 – Global Survey: Telecoms Survey, 2020
6 V. D. Blondel et al., “Data for Development: the D4D Challenge on Mobile Phone Data,” arXiv:1210.0137 [physics, stat], Jan. 2013, Accessed: Jan. 28, 2021. [Online]. Available: http://arxiv.org/abs/1210.0137.
Extract from our white paper : Challenges and advancements in the era of data and artificial intelligence